Why the potential to save lives outweighs the perceived threats
AI is a top priority for healthcare, with a focus on cloud and edge solutions. However, infrastructure challenges persist.
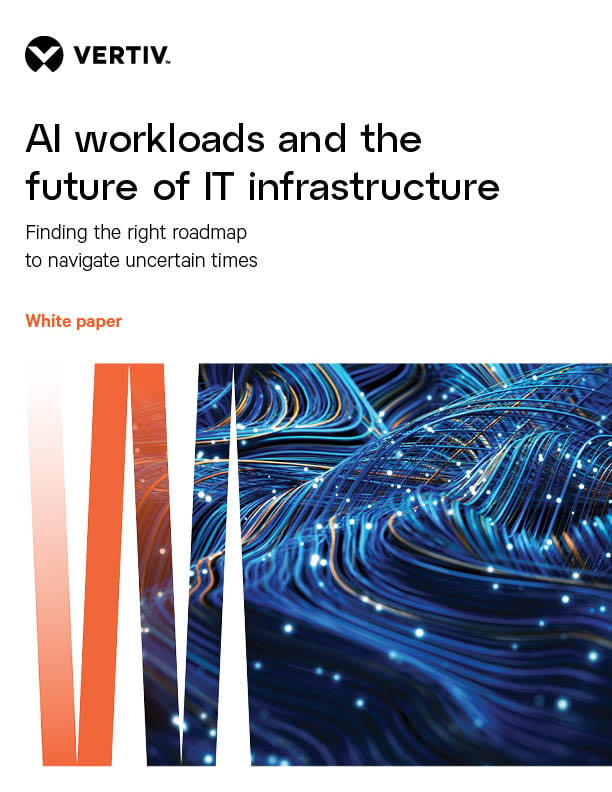
From fears about the safety of autonomous vehicles to rogue military drones, concerns persist about AI being a risk to life. However, as the debate around AI matures, a more nuanced discussion is being had about its potential to also save lives and improve healthcare outcomes.
AI in healthcare is advancing rapidly, driven by its potential to improve patient outcomes, care experiences, clinician well-being, and cost-efficiency—the pillars of the quadruple aim. The global AI in healthcare market is projected to grow from USD 14.92 billion in 2024 to 164.16 billion by 2030, at a CAGR of 49.1%, with PwC’s 2023 Emerging Technology Survey naming AI the top priority for health services’ technology investments.
Global initiatives, including U.S. Department of Health and Human Services (HHS) guidelines, the World Health Organization’s (WHO) responsible AI framework, and the European Union’s (EU) Artificial Intelligence Act, are supporting AI adoption by establishing ethical standards for patient safety, data privacy, and accountability. However, realizing AI’s transformative potential requires robust infrastructure to meet its growing computational and operational demands.
Cloud vs Edge infrastructure
Cloud computing in healthcare provides organizations with scalable storage and advanced AI training capabilities, enabling the management of large datasets and complex AI models. According to a Global Healthcare Exchange (GHX) survey, nearly 70% of U.S. health systems and hospitals plan to adopt cloud-based solutions for supply chain management by 2026, highlighting the growing reliance on cloud infrastructure.
Similarly, edge computing is gaining traction as AI applications demand higher computing density and power efficiency, requiring advanced cooling and resilient infrastructure. With the global edge IT infrastructure market projected to reach $317 billion by 2026 and IoT devices expected to grow to 29 billion by 2030, edge computing is becoming a critical enabler of healthcare innovations like remote monitoring and AI-driven diagnostics.
$164b
is the projected size of the global AI in healthcare market by 2030, up from $14.92 billion in 2024, with a CAGR of 49.1%.
77%
of healthcare stakeholders prioritize AI in emerging tech investments, a PwC survey shows.
70%
of U.S. health systems and hospitals plan to implement cloud-based supply chain management solutions by 2026, a GHX survey shows.
AI imperatives in healthcare
The choice between cloud and edge depends on the specific use case, with cloud offering flexibility and scalability, while edge enables efficiency and responsiveness. As the table shows, data center technologies align with key AI imperatives in healthcare, connecting use cases to the infrastructure required for optimal performance.
Imperative | Use case examples | Data center requirement |
---|---|---|
Be transformative | Drug discovery and testing. Disease detection and treatment. |
Cross-functional expertise. Holistic solution design. |
Be efficient | Automated scheduling, billing, and claims processing. | Extend value and function of existing systems. Close-coupled systems that minimize space and energy requirements. |
Be first | Drug discovery and testing. Disease detection and treatment. |
Designs that reduce time and risk. Pre-configured systems that reduce deployment times. |
Be confident | Enhanced diagnostics and imaging. Accuracy and completeness of clinical documentation. |
Proven solutions supported by comprehensive services. |
Be future-ready | Precision medicine and personalized treatments. Holographic and hybrid imaging. Digital twins in healthcare. Immunomics and synthetic biology. |
Interoperable, upgradable, and scalable solutions that can adapt to change. |
AI use cases in healthcare
AI can alleviate strain across the healthcare industry, benefiting patients, payers, and healthcare professionals, with the following examples:
- Administrative efficiency: AI can save about 20% of doctors’ time by reducing paperwork, allowing more time for patient care. At Johns Hopkins Hospital, AI improved patient flow with 38% faster bed assignments for emergency department admissions. By 2025, AI-driven efficiency could cut healthcare costs by USD 13 billion through fewer errors.
- Preventive care: Machine learning (ML) algorithms analyzed medical images to identify patterns and anomalies, enabling early disease detection with up to 93% accuracy. Mayo Clinic researchers found that kidney size correlates with the rate of function decline, helping detect predisposition to polycystic kidney disease (PKD) and plan prevention.
- Drug discovery: MIT researchers used AI to screen over 100 million chemical compounds, leading to the development of Halicin , an effective antibiotic against many drug-resistant bacteria strains. Biotech company Recursion identified over 20 investigational new drugs using AI, several of which are now in clinical trials. AI can reduce research time and costs by 25-50%, potentially reaching USD 26 billion annually in savings.
- Remote monitoring and telehealth: AI-enabled remote patient monitoring systems (RPM) can achieve 99.62% accuracy for atrial fibrillation detection and 98% for fall detection. Telehealth can handle 70% of non-emergency cases in clinical settings, reducing healthcare professionals’ workload and expanding remote diagnostics, especially in rural areas.
- Surgical assistance: AI-guided robotic systems enhance precision, reduce complications, and improve recovery times in minimally invasive surgeries. For example, a study reported that AI can make the detention of tumor cells 20% more sensitive.
Concerns about AI being a risk to life will no doubt persist, but given the breadth of opportunity to further advance healthcare technology, the positives appear to outweigh the perceived threats. The real challenge for healthcare IT organizations will be understanding where and how to invest in AI technologies and related data center infrastructure to make the most of AI’s potential to be a life-saving technology.
Key takeaways:
AI growth
AI is improving efficiencies in
healthcare, with rapid adoption across diagnostics, treatment, and operations.
Cloud vs Edge
Cloud scales AI for diagnostics; Edge enables real-time monitoring. Hybrid balances both.
AI infrastructure
Scalable, interoperable, and energy-efficient systems power diagnostics, operations, and future-ready care.